our insight
AI tips & trends
Join CX, product, ops, and engineering leaders worldwide that receive The Signal - Sturdy's Blog Newsletter.
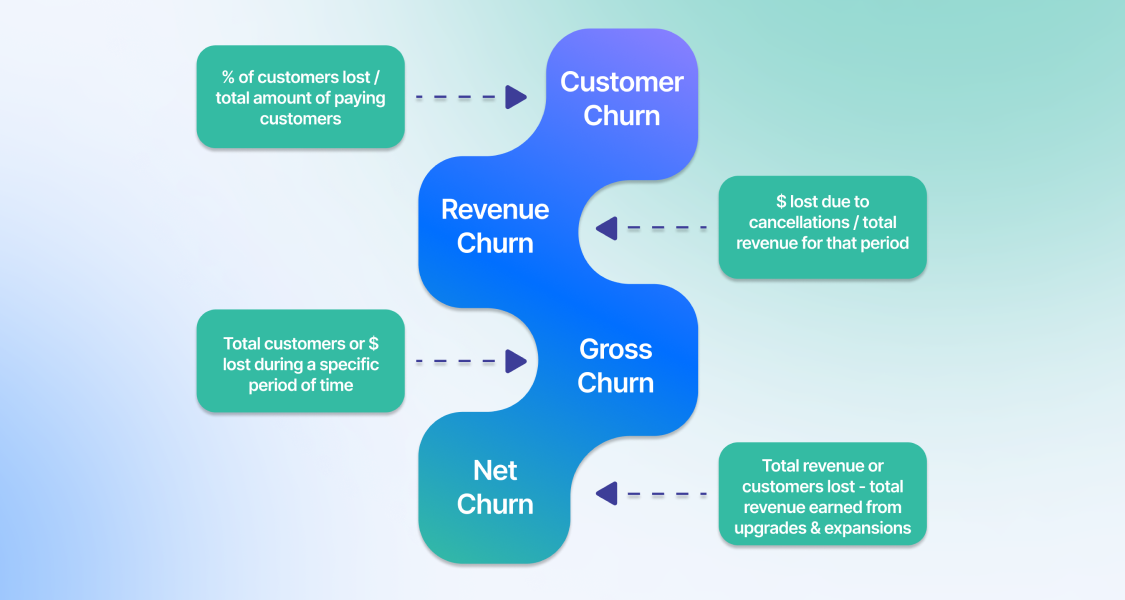
The four types of SaaS churn and how to calculate them
Customer churn is a term often used in the SaaS world, but what does it actually mean?
Simply put, churn is the rate at which customers are lost. These are customers that have canceled your service and aren’t coming back. It can be calculated for individual customers (B2C) or for an entire company (B2B). Four different types of churn are commonly measured: customer churn, revenue churn, gross churn rate, and net churn rate. Let's take a closer look at each type.

Customer Churn
Customer churn is the most commonly used type of churn. It is the percentage of customers that stopped using your company's products or services during a specific time frame. You can calculate your customer churn rate by dividing the number of customers you lost during that period — say a quarter — by the number of customers you had at the beginning of that period.
Let’s pretend for a moment that you work on the growth team at SaaS.io, a new (you guessed it) SaaS startup. Over the last few months, SaaS.io has continued to grow hand over fist with little to no customer churn. However, customer acquisition has begun to slow, and your boss is asking you to calculate the customer churn rate in October. This equation is relatively straightforward. At the beginning of October, Saas.io had 54 customers. However, by the end of the month, two had churned. That means your customer churn rate in the month of October was 3.7%.
1. Total customers at the beginning of a period: 54
2. Number of customers lost in period: 2
3. Customer Churn Rate = (2/54)*100 = 3.7% (that is a great number, by the way)

Revenue Churn
Revenue churn is similar to customer churn, but instead of measuring customers leaving the company, it measures the amount of revenue lost due to customers who have left or downgraded their plans. To calculate revenue churn, divide the total amount of revenue lost in a certain period by the total revenue at the beginning of that period.
If we head back to our SaaS.io example, it’s important to note that the October revenue churn is much scarier than the customer churn. Yes, only two customers churned, meaning there was a 3.7% customer churn rate. However, one of those customers (Customer 2) accounted for 11% of MRR (monthly recurring revenue). Customer 1 generated only $6,000 in MRR, whereas Customer 2 generated $22,000 MRR. That means that at the beginning of October, SaaS.io’s MRR was $200,000. By the end of October, the revenue churn was .14.
1. Total revenue at the beginning of a period: $200,000
2. Net revenue lost in period: $6,000 + $22,000 = $28,000
3. Revenue Churn Rate = $28,000/$200,000 = .14
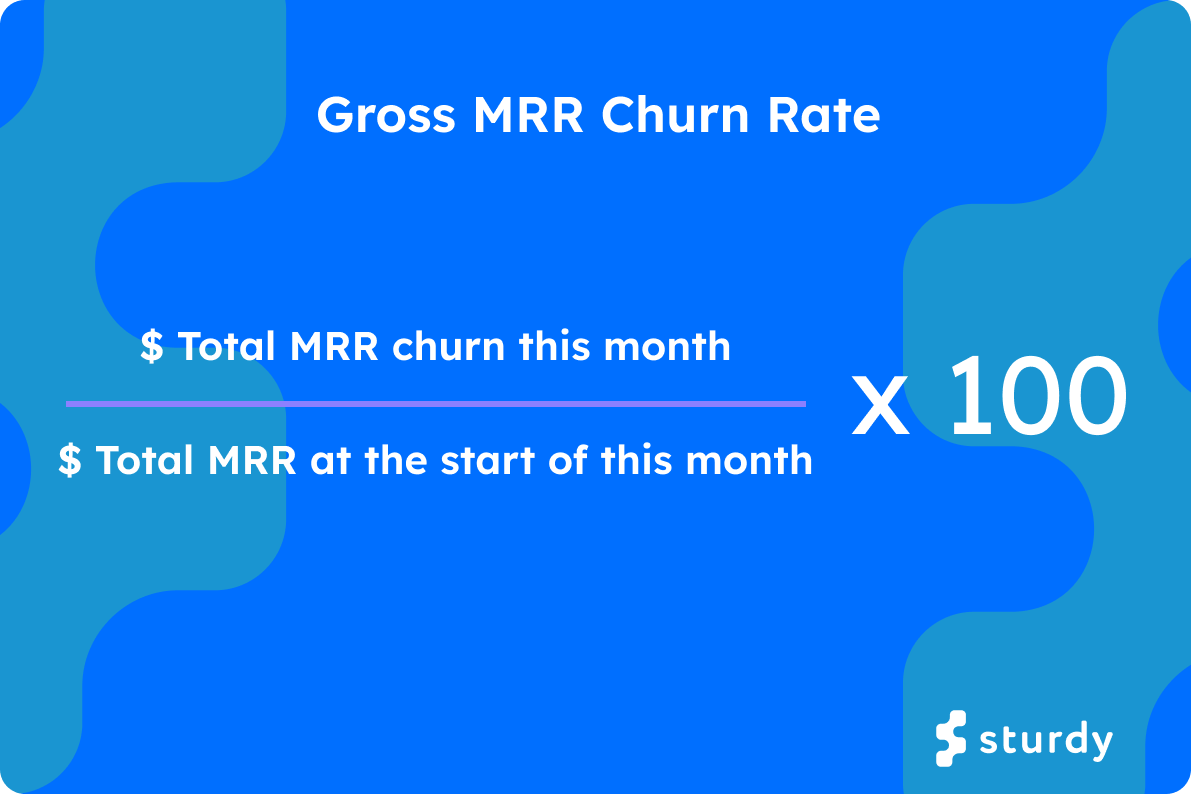
Gross Churn Rate
The Gross churn rate takes into account both customer and revenue churn. It measures the total number of customers and revenue lost in a certain period, divided by the total number of customers and revenue at the beginning. This gives an overall picture of how much business is lost in a given time frame.
If we apply this to SaaS.io, the MRR for October was $200,000, and users canceled $28,000 worth of contracts. That means the gross churn rate will be 14%
1. Total revenue at the beginning of a period: $200,000
2. Net revenue lost in period: $6,000 + $22,000 = $28,000
3. Gross Churn Rate = ($28,000/$200,000) x 100% = 14%
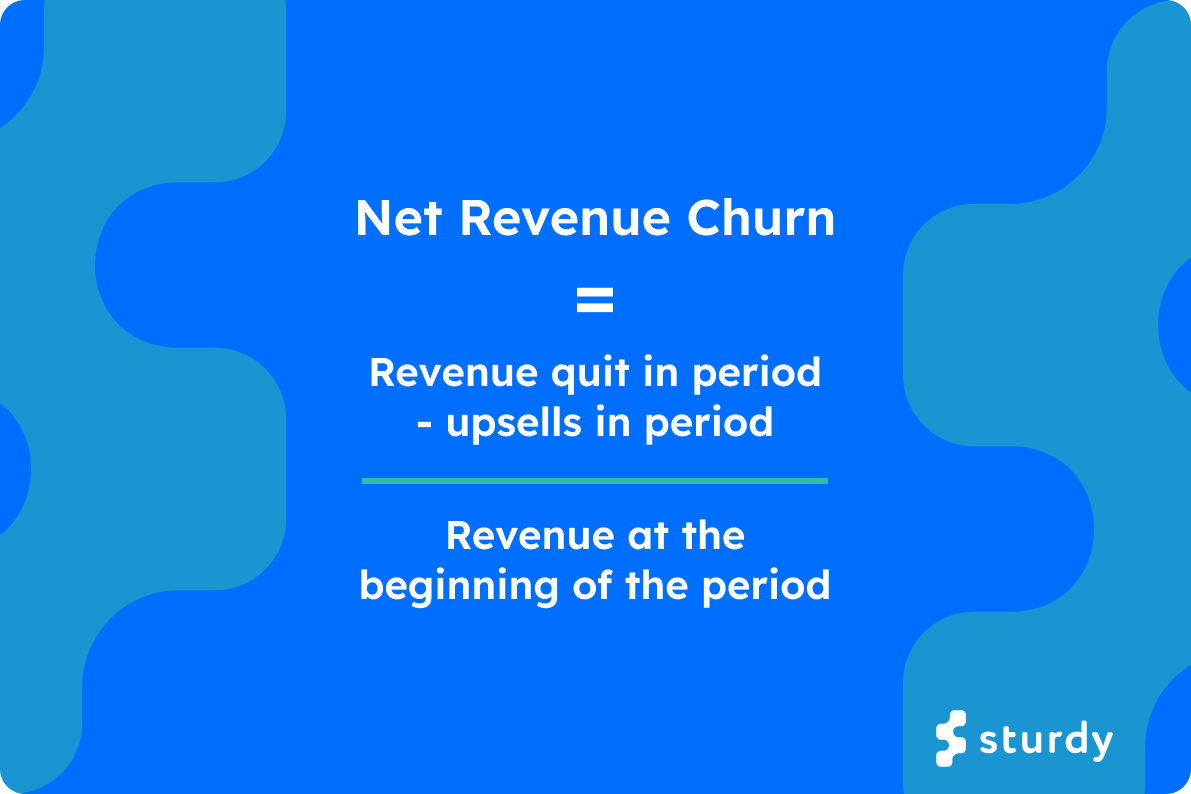
Net Churn Rate
Net churn rate considers both customer and revenue churn. However, it also includes new customers and expansion revenue acquired in a certain period. Expansion revenue is the additional revenue you generate from existing customers through upsells, cross-sells, or add-ons. That’s why net revenue churn gives an overall picture of how much business is being gained or lost in a given time frame.
A month has passed since those two customers, and 14% of gross MRR was lost. Saas.io is currently at $172,000 MRR in November, as no additional sales have been made. Unfortunately, November has also seen $12,000 in contract losses. Luckily for Saas.io, a few existing customers have upgraded their plans, generating an additional $10,000 in revenue. Your boss asks you what the net churn rate for November is. First, you must subtract the customer upgrade revenue from the revenue lost in downgrades and cancellations. Then, divide that number by the revenue at the beginning of November.
1. Total revenue at the beginning of a period: $172,000
2. Net revenue lost in period: $12,000 - $10,000 = $2,000
3. Net Churn Rate = $2,000/$172,000 = 1.1%

Leaky Bucket Equation
At the beginning of this post, we noted that four types of churn could be measured. That isn’t entirely true, so here’s a bit of a bonus round. SaaS angel investor, Dave Kellogg argues that the leaky bucket equation “should always be the first four lines of any SaaS company’s financial statements.” Kellogg continues, “I conceptualize SaaS companies as leaky buckets full of annual recurring revenue (ARR). Every time period, the sales organization pours more ARR into the bucket, and the customer success (CS) organization tries to prevent water from leaking out”.
Kellogg defines the leaky bucket equation as “Starting ARR + new ARR - churn ARR = ending ARR”.
If we apply this to our Saas.io example, we can determine that the starting ARR in the fourth quarter (Q4) of 2022 was roughly $400,000. The new ARR in Q4 ‘22 was $56,000, and the Churn ARR in that same time period was $45,000. In other words:
1. Total starting ARR: $400,000
2. New ARR: $56,000 & Churn ARR: $45,000
3. Ending ARR = $400,000 + $54,000 - $45,000 = $409,000
Churn is an important metric to track for any SaaS company, as it can be used to identify trends, measure loyalty, and assess the effectiveness of customer retention strategies. Calculating churn rates can help companies identify which customers are more likely to leave and which types of customers are the most valuable. By understanding churn, businesses can take steps to improve customer retention and keep their business running smoothly.
Customer churn is a term often used in the SaaS world, but what does it actually mean?
Simply put, churn is the rate at which customers are lost. These are customers that have canceled your service and aren’t coming back. It can be calculated for individual customers (B2C) or for an entire company (B2B). Four different types of churn are commonly measured: customer churn, revenue churn, gross churn rate, and net churn rate. Let's take a closer look at each type.

Customer Churn
Customer churn is the most commonly used type of churn. It is the percentage of customers that stopped using your company's products or services during a specific time frame. You can calculate your customer churn rate by dividing the number of customers you lost during that period — say a quarter — by the number of customers you had at the beginning of that period.
Let’s pretend for a moment that you work on the growth team at SaaS.io, a new (you guessed it) SaaS startup. Over the last few months, SaaS.io has continued to grow hand over fist with little to no customer churn. However, customer acquisition has begun to slow, and your boss is asking you to calculate the customer churn rate in October. This equation is relatively straightforward. At the beginning of October, Saas.io had 54 customers. However, by the end of the month, two had churned. That means your customer churn rate in the month of October was 3.7%.
1. Total customers at the beginning of a period: 54
2. Number of customers lost in period: 2
3. Customer Churn Rate = (2/54)*100 = 3.7% (that is a great number, by the way)

Revenue Churn
Revenue churn is similar to customer churn, but instead of measuring customers leaving the company, it measures the amount of revenue lost due to customers who have left or downgraded their plans. To calculate revenue churn, divide the total amount of revenue lost in a certain period by the total revenue at the beginning of that period.
If we head back to our SaaS.io example, it’s important to note that the October revenue churn is much scarier than the customer churn. Yes, only two customers churned, meaning there was a 3.7% customer churn rate. However, one of those customers (Customer 2) accounted for 11% of MRR (monthly recurring revenue). Customer 1 generated only $6,000 in MRR, whereas Customer 2 generated $22,000 MRR. That means that at the beginning of October, SaaS.io’s MRR was $200,000. By the end of October, the revenue churn was .14.
1. Total revenue at the beginning of a period: $200,000
2. Net revenue lost in period: $6,000 + $22,000 = $28,000
3. Revenue Churn Rate = $28,000/$200,000 = .14
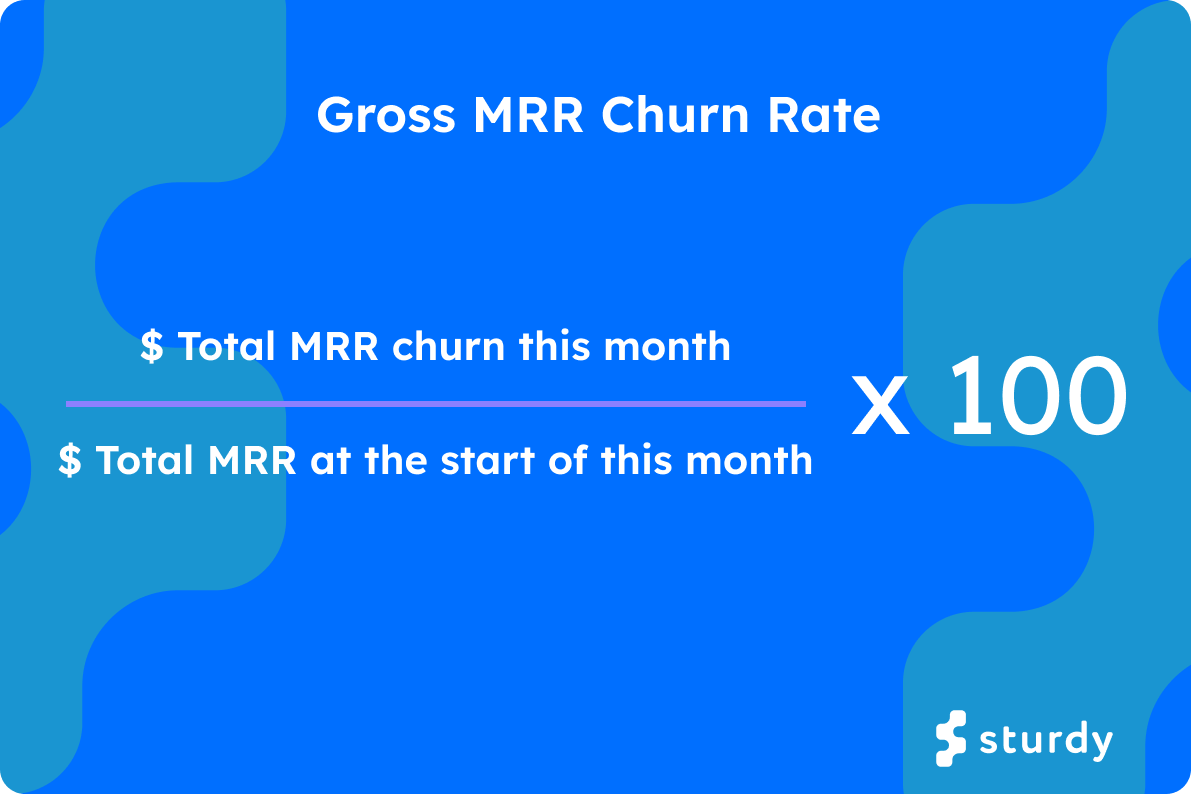
Gross Churn Rate
The Gross churn rate takes into account both customer and revenue churn. It measures the total number of customers and revenue lost in a certain period, divided by the total number of customers and revenue at the beginning. This gives an overall picture of how much business is lost in a given time frame.
If we apply this to SaaS.io, the MRR for October was $200,000, and users canceled $28,000 worth of contracts. That means the gross churn rate will be 14%
1. Total revenue at the beginning of a period: $200,000
2. Net revenue lost in period: $6,000 + $22,000 = $28,000
3. Gross Churn Rate = ($28,000/$200,000) x 100% = 14%
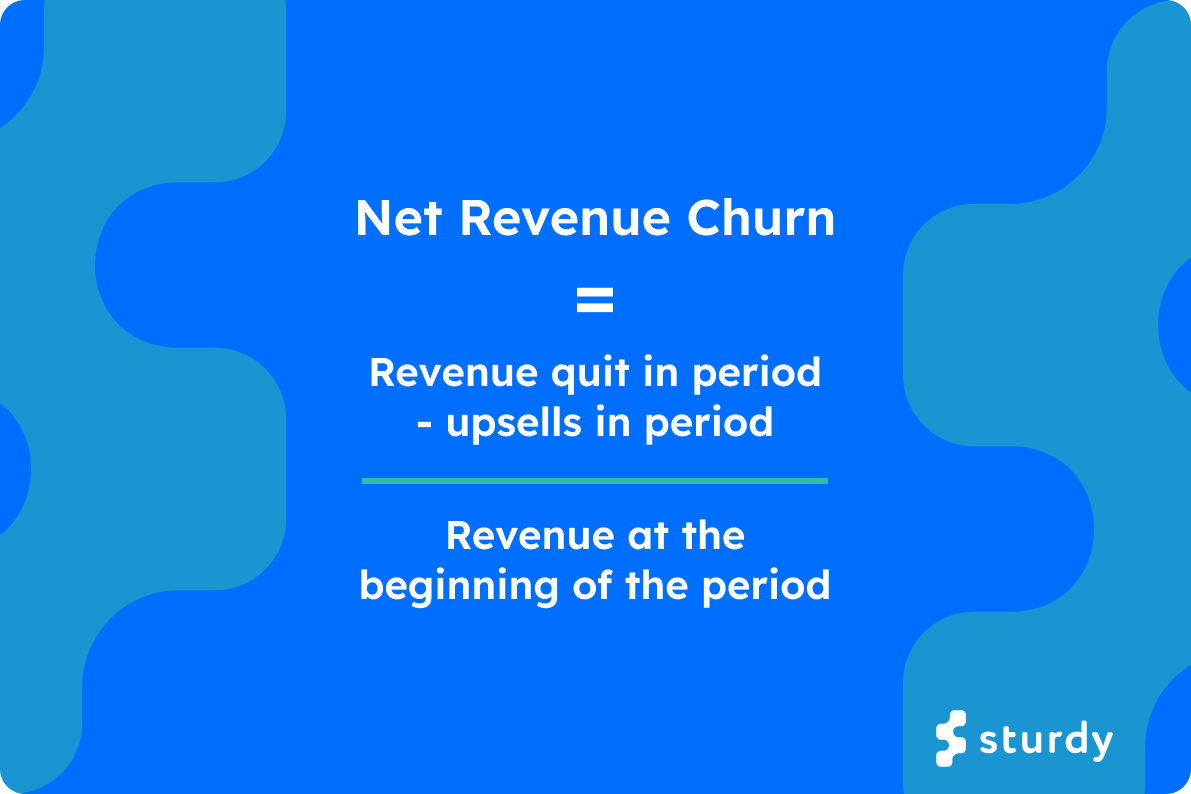
Net Churn Rate
Net churn rate considers both customer and revenue churn. However, it also includes new customers and expansion revenue acquired in a certain period. Expansion revenue is the additional revenue you generate from existing customers through upsells, cross-sells, or add-ons. That’s why net revenue churn gives an overall picture of how much business is being gained or lost in a given time frame.
A month has passed since those two customers, and 14% of gross MRR was lost. Saas.io is currently at $172,000 MRR in November, as no additional sales have been made. Unfortunately, November has also seen $12,000 in contract losses. Luckily for Saas.io, a few existing customers have upgraded their plans, generating an additional $10,000 in revenue. Your boss asks you what the net churn rate for November is. First, you must subtract the customer upgrade revenue from the revenue lost in downgrades and cancellations. Then, divide that number by the revenue at the beginning of November.
1. Total revenue at the beginning of a period: $172,000
2. Net revenue lost in period: $12,000 - $10,000 = $2,000
3. Net Churn Rate = $2,000/$172,000 = 1.1%

Leaky Bucket Equation
At the beginning of this post, we noted that four types of churn could be measured. That isn’t entirely true, so here’s a bit of a bonus round. SaaS angel investor, Dave Kellogg argues that the leaky bucket equation “should always be the first four lines of any SaaS company’s financial statements.” Kellogg continues, “I conceptualize SaaS companies as leaky buckets full of annual recurring revenue (ARR). Every time period, the sales organization pours more ARR into the bucket, and the customer success (CS) organization tries to prevent water from leaking out”.
Kellogg defines the leaky bucket equation as “Starting ARR + new ARR - churn ARR = ending ARR”.
If we apply this to our Saas.io example, we can determine that the starting ARR in the fourth quarter (Q4) of 2022 was roughly $400,000. The new ARR in Q4 ‘22 was $56,000, and the Churn ARR in that same time period was $45,000. In other words:
1. Total starting ARR: $400,000
2. New ARR: $56,000 & Churn ARR: $45,000
3. Ending ARR = $400,000 + $54,000 - $45,000 = $409,000
Churn is an important metric to track for any SaaS company, as it can be used to identify trends, measure loyalty, and assess the effectiveness of customer retention strategies. Calculating churn rates can help companies identify which customers are more likely to leave and which types of customers are the most valuable. By understanding churn, businesses can take steps to improve customer retention and keep their business running smoothly.
Our articles
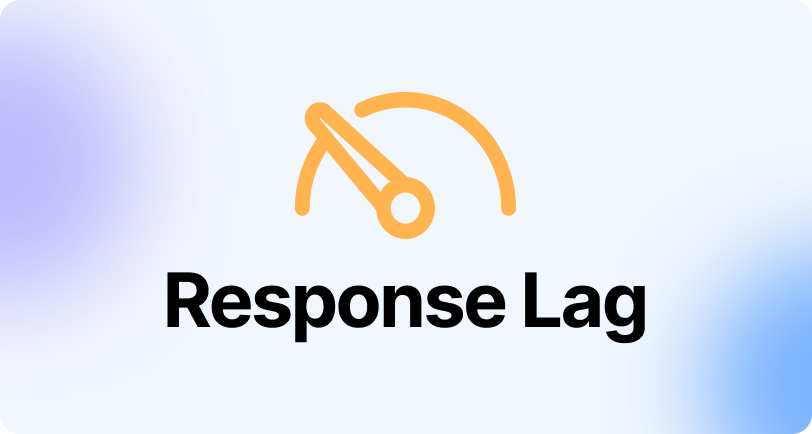
The Scary Six: Response Lag
I was speaking to the COO of one of our customers a few weeks back, and he said that Sturdy’s “Response Lag” signal was his “Laptop Smasher.” This signal is defined as a “customer is asking for a status update on an unresolved issue.” If your goal is to make sure your customers feel heard, then it is a bad one.
While not AI-based, the attached regex will help you find some of these messages on your own. If your support or BI system allows you to filter on inbound messages it will provide cleaner results. (There’s quite a bit of contextual difference between a customer asking for an update and one of your people asking a customer for an update).
In most cases, this signal is pretty rare. Typically, it occurs about once per every 1,000 conversations (again, only detecting messages “coming in” from a customer).This signal is important to track for two reasons. The first is that it is almost never self-reported. It is rare for a customer-facing person to say, “Yeah, the customer is upset because I never got back to them.” I am almost certain that you have CS/Support teammates who have a much higher incidence of this signal than your best performers. It also means that you have a grumpy customer that you don’t know about.
The second reason is that Response Lags provide really good data for resolving hidden process or product gaps. If a customer is asking for an update on an issue, it is likely that several other customers are asking about the same thing. Every business is different, but Response Lags will likely indicate that there is a product, process, or person responsible for the plurality of them.
At Sturdy, we use machine learning to track, record, and alert you of Response Lags. We’re also working on some cool stuff that will track the response time of any open issue from any conversation (without requiring a customer to hit the “is this resolved?” button).
How cool would it be to have a dashboard of every “waiting for a response,” email, chat or phone call? We’re working on it.
Give the regex a try, and feel free to DM me with any questions. I hope you don’t smash a laptop. Of course, please regale us in the comments of any learnings you’d like to share on the subject.
Do that hard things,
Steve
.png)
The Scary Six: Executive Change
At the end of last year, I shared a regular expression (regex) that identifies "contract requests." That's a scary signal for people who like to keep customers.
Today, I want to discuss the scariest of the Scary Six, "Executive Change."
At my last company, Newton, this signal had the highest correlation to churn and initially resulted in a loss about 50% of the time (for many of Sturdy's customers, this is also true).
So what is it? Let's say you sell accounting services, and this happens:
"Hi, I am the new CFO, and I would like a quick rundown of your capabilities."
The response is often,
"So nice to meet you! LMK when you have 30 minutes for a quick call!"
(By the way, usage will be high during this time, and their Health Score will be green.)
On to the regex…
The first two are specific to HR services/tech, so replace "hr" with "e-commerce," "accounting," "logistics," or whatever business you're in.
Here's what they do:
1. The first detects when someone says, "Hey, we have a new VP of HR coming on board soon."
2. The second, "I will be taking over the Admin role for this account."
3. The third, "Hey, I wanted to let you know that I will be leaving at the end of January."
Remember that they return a fair number of false positives (FPs). FPs are not included in the churn rate calculation.
The frequency of "Executive Change" varies depending on the industry and segment. In the SMB cohort, it occurs in about .1% to .2% of customer conversations. In huge enterprises, around .04%.
Interestingly, this signal is much more common in the HR space, firing at .3% per conversation.
There is also a lot of variation in the severity. Still, the correlation to cancellation is the 2nd highest of any signal we currently detect at Sturdy ("I want to cancel" being the highest, obviously). For SMB customers, the churn rate for this signal, if untreated, will approach 70%. It will be lower for enterprise customers.
Another critical point is that this is a leading indicator. It often occurs long before the cancellation event.
Why is this signal such a strong indicator? At the beginning of the post, we showed a sample trigger-sequence that ended something like, "Let's do a quick demo!"
What's wrong here? I think it is because one or all of the following is happening:
1. The value of your service can't be communicated in a "quick demo."
2. The new contact has undoubtedly used and trusts a competing solution.
3. The person conducting the demo has not been trained to sell your product, overcome objections, and destroy your competition's product.
This is a perfect recipe for failure. Here's a scenario...
Acme Corp sells HR Software on M2M and yearly contracts; it receives:
10k emails and tickets per month (items).
10k items equals to about 2k conversations (1 convo = ~ 4.4 items)
.3% detection per conversation = 6 Exec Changes
Two false-positive (30% FP rate)
50% churn x 4 = 2 losses
If untreated, Acme loses two customers to this signal per month.
The good news is that, in my experience, treatment will save about one of these customers each month. How?
1. Train everyone who touches customers, billing, CS, and marketing to identify the signal.
2. Immediately send the signal to your sales AND marketing teams.
Someone should attempt to discover the product the new contact used at their former company.
3. A salesperson must schedule a demo as soon as possible. (At Newton, our KPI was to conduct the demo within ten days). The seller should come armed with useful information, like usage data candidates hired (e.g.), and be prepared to sell against the new contact's previous solution.
4. In parallel, the marketing team checks LinkedIn to see if the previous contact has landed a new job. If not, someone should reach out and see if they need help in their job search (after all, you sell to companies that hire these people). If the person has landed somewhere, send them a note, a gift basket, or whatever you think is appropriate.
5. Send the previous contact to the Sales team as an SQL.
(Shameless plug: Sturdy has AI-language models that find 1, automatically route 2, and can tell you if 3 and 4 happened.)
The result of this process is a successful "double-dip". You may save a customer and gain a lead for your sales team. Ironically, if your competition is not tracking the Executive Change signal, your chance of closing that deal is very high.
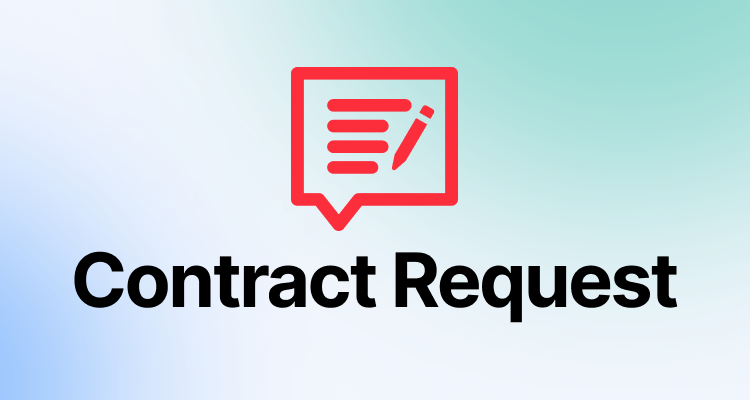
The Scary Six: Contract Request
The second line of that image is a regular expression (aka regex). If your support or ticket system supports regex, try that search against the content of your tickets. You can probably hand this to your BI team, too. It will find customer comments like, “Hey, we’re just cleaning up some files, and can we get a copy of our agreement?”
For some background, at my last company, I had a standing meeting on my calendar every week to read random support tickets. From this, the concept of the “Scary Six” was born.
One of the Scary Six was a “Contract Request.”
At Newton, about 70% of the time, when a customer requested a copy of their contract, it was a risk to their revenue longevity. We audited them regularly and found they broke down into the following buckets:
We want to know when we can or how easy it is to cancel (50%).
We just need our contract because we lost it (30%).
We are getting bought, going out of business, etc. (10%).
We need to see if we can cut some costs (10%).
We saw this flag about once per 6,000 email conversations (.0167%). Generally, this average rings true for most businesses we work with today.
Combining these two metrics, we estimated that for every 10,000 email conversations, we received about 2 Contract Requests. In other words, for every 10,000 emails, we had 1.4 customers at risk.
Once we identified Contract Request as a revenue impact, our incredible CS team trained everyone to identify “Contract Request” language. We then built a process for addressing them.
The before/after impact of identification and triage was remarkable and resulted in doubling the retention rate for this signal.
Over the next few weeks, I will post the rest of the “Scary Six” with their regex. Those left on the list are “Executive Change,” “Renewal,” “Response Lag,” “Overpromised,” and obviously, “Cancellation.”
Please let me know if you have any other “Scary” triggers. I hope you give this a shot and find it illuminating.
.avif)
There's a New Sheriff in Town
When I started my first SaaS company, I had a standing meeting on my calendars every week to read random support tickets (random is the crucial word, by the way). Reading tickets was always illuminating and often painful. One of our learnings was a churn risk called "New Sheriff."
First, don't get me wrong, we trusted our team. But if there's one thing that always bothered me, I never really knew what our customers said about us. And, for that matter, what we were saying to them.
Eventually, we built a suite of search strings, and if you want to try some yourself, here are a few simple ones:
We would search for product issues with things like: "doesn't work"; "confusing"; "annoying" bug, and "clear cache."
Searching for things like "gotten back to me" and "still waiting" would indicate that our customer was still awaiting a response. I would look for revenue issues with: "new VP,"; "new vice president,"; "new manager,"; "has left the company,"; "copy of our contract,"; "renewal date," and "overdue."
You are probably thinking, "Why would I look for "new VP" or "new manager"?" It comes up like this, "Our HR Manager has left the company recently, and I need a login for our VP of HR, Jim Smith."
At Newton, HR executives were responsible for hiring/firing HR software decisions. We sold HR software.
A new HR executive was the highest indicator of churn in our business. By that, I mean, left unattended, our customer was almost certainly (80%+) going to churn at renewal. From this, the term "New Sheriff" was coined. A "New Sheriff" customer was no longer forecasted to be a long-term customer and thus needed to be resold.
We trained everyone at Newton on identifying a "New Sheriff" and where to send the alert - manually.
When we got a "New Sheriff" alert, several people got to work. The CS team would pull usage data and some other vital metrics. The account management team would reach out to identify the new VP and schedule a demo of our solution.
Our sales leadership would also reach out to the former executive. We'd offer to help them network to find a new job or make inroads at their new company.
In doing this, we turned our "churniest" event, one with an 80% churn rate, to one with a 30% churn rate (from -.8 to -.3). We also gained a lead for our sales team that closed 80% of the time (from 0 to +.8). In other words, we turned a very churny event into one that gained a half a customer.
If you'd like to capture "New Sheriffs," give me a shout, and I'll send you a few more advanced search strings. (If you’re a Sturdy customer, our models auto-flag this as “Executive Change.”)- Steve@sturdy.ai
.png)
The Rise of AI Operations Management
About the Author
Hi, I'm Steve Hazelton. I am one of the founders of a startup that helps businesses better understand their customers by using AI to identify risks and opportunities inside the unstructured data trapped in emails, chats, and phone calls. I received help writing this article by using generative AI for data collection (if you don’t know what that means, you are not alone, go here).
Caveat Emptor: Since I am the founder of a startup that relies heavily on AI to find happy and unhappy customers, I certainly have "bought in" to using AI for business to leverage untapped data streams. So, consider yourself warned. I also started my career a long time ago as a recruiter for technology companies, later built and sold an HR tech company, and later started an AI company…so the intersection of AI and career advice collide here.
Introduction
Often lost in this discussion of AI technology is the discussion of its impacts on our teammates and coworkers. What does the widespread adoption of AI in business mean for the careers of people who work at these businesses? While many of us are, and should be, concerned about job destruction, I want to talk about job creation.
Note: There is, at present, endless discussion on AI technology, which I won't bore you with here. If you want to learn about the different types of AI and AI tools, like Generative AI, Synth AI, Machine Learning, and others, you can read an article our co-founder wrote here.
From a career standpoint, the most significant change our businesses will see this decade is creating a new, high-paying job in AI Operations. This article will help you, the reader, define this role when you decide to hire this person, or if you desire to be that person, how to create the role in your company.
This person will leverage AI tools and products to improve a business's top and bottom-line revenue. They'll find revenue opportunities, prevent cancellations or churn, and make people more efficient. They will be indispensable.
Dan Corbin, an instructor at the Pragmatic Institute, states, "If you can change your mindset as a company and understand the capabilities of AI, this is where AI operations come in. You need this AI Operations Director to ask, "How do we tackle this from a macro level?" You must think about AI from an organizational perspective to leverage it to its full capacity."
We are at the beginning of a major, major shift in employment. Fifty years ago, did companies have an IT Manager? No, but they do now. Thirty years ago, did they have an e-commerce manager? Again, no, but they do now. Ten years from now, will companies have someone in charge of AI operations? Of course, they will.
Adoption is inevitable because the gains are too significant. As Mike Evans, Director of Customer Care and Analytics at Laerdal Medical, states, "You need someone to own this." Companies like MassPay have already implemented such a role, which they attribute to the 100% customer retention of their "Top 100" last year. Hawke Media, the top performance marketing agency in the country, shifted the purview of their existing Director of Business Intelligence to include AI Ops. They then improved revenue retention by 30% MoM in less than six weeks.
With that out of the way, let's get started.
The Role of a Director of AI Operations
As businesses continue to embrace AI technologies, the need for dedicated professionals to implement and oversee these systems will become critical. Enter the Director of AI Operations.
At its core, this role is creative: you need to think of new ways to solve old problems in ways that have never been done before. "How can we use tomorrow's tools to solve our problems today?" This is a key point. AI will help you solve problems in ways you never considered before because they were previously impossible.
The Director of AI Operations will be the key player responsible for developing, implementing, and managing AI strategies.
The AI Business Director should be able to create and implement an AI strategy that answers the following:
How can we use AI to find revenue opportunities?”
How can we use AI to identify and reduce revenue risks?”
How can AI make our teammates more efficient?”
This multifaceted role requires a deep understanding of AI vendors, data privacy, and a visionary mindset to leverage AI's potential effectively.
Note: This job does not require coding. This person isn't building AI; they are identifying the areas where AI can improve business performance.
Let's take a closer look at the responsibilities of this job:
Strategy Development: "What problems are we trying to solve?" The Director of AI Operations collaborates with various departments to identify areas where AI can be integrated to capture risk and opportunities or to improve efficiency. They create a comprehensive AI strategy aligned with the business's overall objectives.
Data Discovery: "What data could AI illuminate that we've previously been unable to use?" For example, a Director of AI Operations could use emails to create a new data stream that correlates customer product confusion with unhappiness and eventual cancellation.
Data Management: "What are the security, privacy, and regulatory challenges with our approach." AI heavily relies on quality data to make accurate predictions and decisions. The Director ensures that data is collected, cleaned, and stored securely. This person should be able to deep-dive on a vendor's privacy and regulatory compliance.
Implementation: "What systems will we need to leverage, and how will we accomplish this?" Once the AI strategy is in place, the Director oversees the implementation of AI projects, ensuring seamless integration with existing systems and addressing any technical challenges that arise. Just as important, this person will need to drive the "people-side" integrations and help people leverage these new data streams.
Performance Monitoring: "What are the success criteria?" Monitoring the performance of AI systems is critical. The Director tracks key performance indicators to measure the impact of AI applications and makes adjustments as needed. Critical here is to answer, "Is this driving the desired outcomes?"
Ethical Considerations: "Should we even use AI for this?" Some AI systems handle sensitive data and will replicate previous biases. A crucial question is, "Is the AI making decisions?" If "yes," then much thought should be put into whether or not AI is appropriate.
Growth of AI Jobs
According to a study conducted by the World Economic Forum, AI is estimated to create 58 million new jobs by 2024. This includes a wide range of roles, from data scientists and AI engineers to, of course, Directors of AI Operations. According to HireEZ, one of the world's largest outbound recruiting platforms, demand for AI-related positions has risen by 60% since 2021.
On the flip side of that coin, higher education and e-learning platforms are seeing a surge in interest in AI courses. Pablo Garcia, Content Lead at CXL, the top marketing e-learning platform, states, "CXL saw a much higher interest in AI courses among our students in 2023, with a 785% increase in engagement for the Advanced AI in Marketing course."
As more companies recognize the potential of AI and seek to stay competitive in the market, the demand for AI professionals is set to skyrocket. A survey conducted by Deloitte further reinforces the growing importance of AI in businesses. It revealed that around 61% of surveyed companies have already implemented some form of AI into their operations. That means 61% of respondents are looking to hire a Director of AI Operations if they haven't already. Don't get left behind.
Where to Start
If becoming your company's Director of AI Operations seems daunting, don't be discouraged if you don't have experience. Very, very few people do. Get started now, and you'll be far ahead of everyone else.
Where would I start? I would look at my current role and think, "How could AI help my current company keep customers longer? Or, how could AI make my group more efficient?"
What problems are we trying to solve?”
What data could AI illuminate that we’ve previously been unable to use?”
What are the security, privacy, and regulatory challenges with our approach?”
What systems will we need to leverage, and how will we accomplish this?”
What are the success criteria?”
Should we even use AI for this?”
Get on it! Also, if you’d like more help, download our AI Retention Plan & Calculator.
Are you looking to hire someone to manage AI Operations?
While writing this article, a friend sent me a job description for a “Head of AI Product Management” at a significant online streaming company. It pays 900k/year. Hmmm… A recent Sturdy poll on LinkedIn concluded that over 50% of respondents already have someone in an AI Operations position, with 25% looking to fill the role in 2024. If you’re interested in hiring for a Director of AI Operations, feel free to copy and paste the job description below:
Director of AI Operations
Role Overview: The Director of AI Operations will be the key player responsible for developing, implementing, and managing AI strategies. This multifaceted role requires a deep understanding of AI vendors, data privacy, and a visionary mindset to leverage AI's potential effectively.
Key Responsibilities:
- The Director of AI Operations collaborates with various departments to identify areas where AI can be integrated to capture risk and opportunities or to improve efficiency. They create a comprehensive AI strategy aligned with the business's overall objectives.
- Identify areas of improvement throughout the business and implement AI workflows.
- The Director ensures that data is collected, cleaned, and stored securely. This person should be able to deep-dive on a vendor's privacy and regulatory compliance.
- The Director tracks key performance indicators to measure the impact of AI applications and makes adjustments as needed.
- Once the AI strategy is in place, the Director oversees the implementation of AI projects, ensuring seamless integration with existing systems and addressing any technical challenges that arise.
- Stay updated with the latest generative and synthesis AI trends and technologies to ensure the company stays ahead of the curve.
- Develop AI strategy and roadmap and act as the foremost thought leader on ethical considerations such as how AI systems handle sensitive data.
- Train colleagues and other teams on AI workflows and best practices for their departments.
Requirements:
- Bachelor’s degree in Computer Science, Data Science, or a related field. Master’s degree preferred.
- Proven experience identifying areas where AI can improve business performance and executing those strategies.
- Strong analytical and problem-solving skills.
- Ability to work collaboratively with diverse teams.
- Excellent communication skills, both written and verbal.
As always, thanks for reading. Feel free to reach out to us to talk further.
Steve
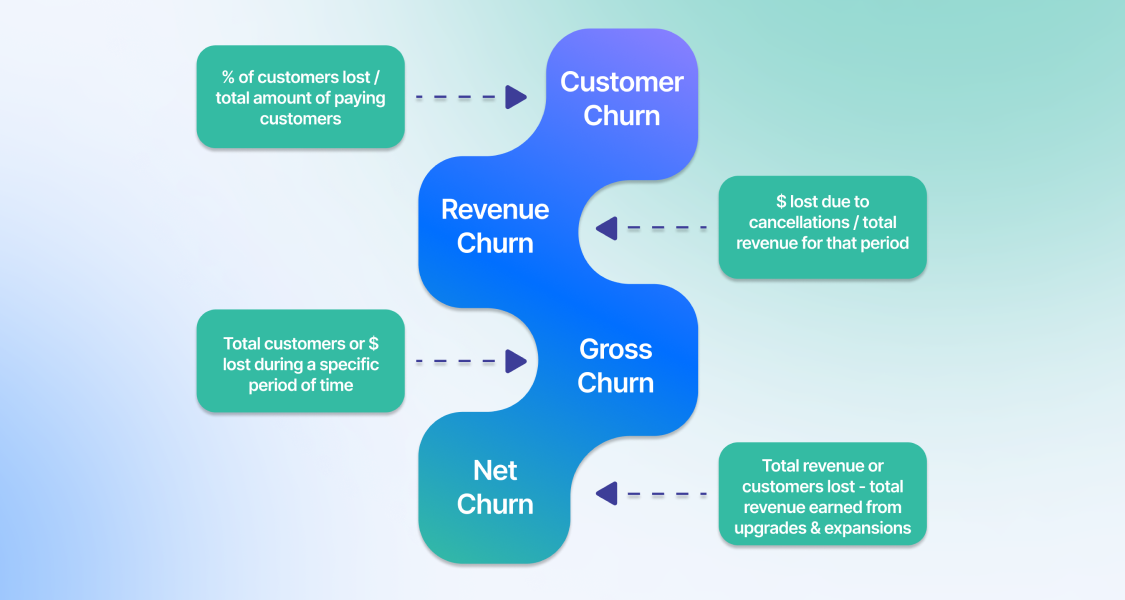
The four types of SaaS churn and how to calculate them
Customer churn is a term often used in the SaaS world, but what does it actually mean?
Simply put, churn is the rate at which customers are lost. These are customers that have canceled your service and aren’t coming back. It can be calculated for individual customers (B2C) or for an entire company (B2B). Four different types of churn are commonly measured: customer churn, revenue churn, gross churn rate, and net churn rate. Let's take a closer look at each type.

Customer Churn
Customer churn is the most commonly used type of churn. It is the percentage of customers that stopped using your company's products or services during a specific time frame. You can calculate your customer churn rate by dividing the number of customers you lost during that period — say a quarter — by the number of customers you had at the beginning of that period.
Let’s pretend for a moment that you work on the growth team at SaaS.io, a new (you guessed it) SaaS startup. Over the last few months, SaaS.io has continued to grow hand over fist with little to no customer churn. However, customer acquisition has begun to slow, and your boss is asking you to calculate the customer churn rate in October. This equation is relatively straightforward. At the beginning of October, Saas.io had 54 customers. However, by the end of the month, two had churned. That means your customer churn rate in the month of October was 3.7%.
1. Total customers at the beginning of a period: 54
2. Number of customers lost in period: 2
3. Customer Churn Rate = (2/54)*100 = 3.7% (that is a great number, by the way)

Revenue Churn
Revenue churn is similar to customer churn, but instead of measuring customers leaving the company, it measures the amount of revenue lost due to customers who have left or downgraded their plans. To calculate revenue churn, divide the total amount of revenue lost in a certain period by the total revenue at the beginning of that period.
If we head back to our SaaS.io example, it’s important to note that the October revenue churn is much scarier than the customer churn. Yes, only two customers churned, meaning there was a 3.7% customer churn rate. However, one of those customers (Customer 2) accounted for 11% of MRR (monthly recurring revenue). Customer 1 generated only $6,000 in MRR, whereas Customer 2 generated $22,000 MRR. That means that at the beginning of October, SaaS.io’s MRR was $200,000. By the end of October, the revenue churn was .14.
1. Total revenue at the beginning of a period: $200,000
2. Net revenue lost in period: $6,000 + $22,000 = $28,000
3. Revenue Churn Rate = $28,000/$200,000 = .14
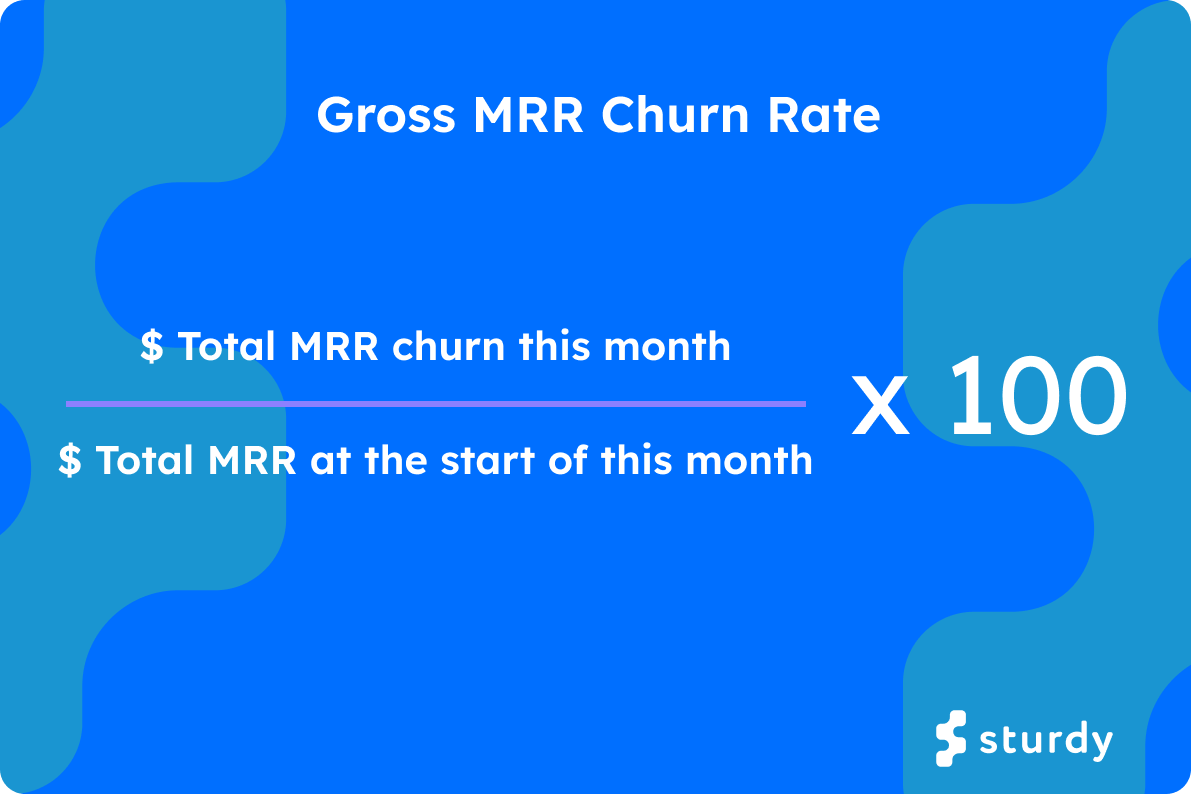
Gross Churn Rate
The Gross churn rate takes into account both customer and revenue churn. It measures the total number of customers and revenue lost in a certain period, divided by the total number of customers and revenue at the beginning. This gives an overall picture of how much business is lost in a given time frame.
If we apply this to SaaS.io, the MRR for October was $200,000, and users canceled $28,000 worth of contracts. That means the gross churn rate will be 14%
1. Total revenue at the beginning of a period: $200,000
2. Net revenue lost in period: $6,000 + $22,000 = $28,000
3. Gross Churn Rate = ($28,000/$200,000) x 100% = 14%
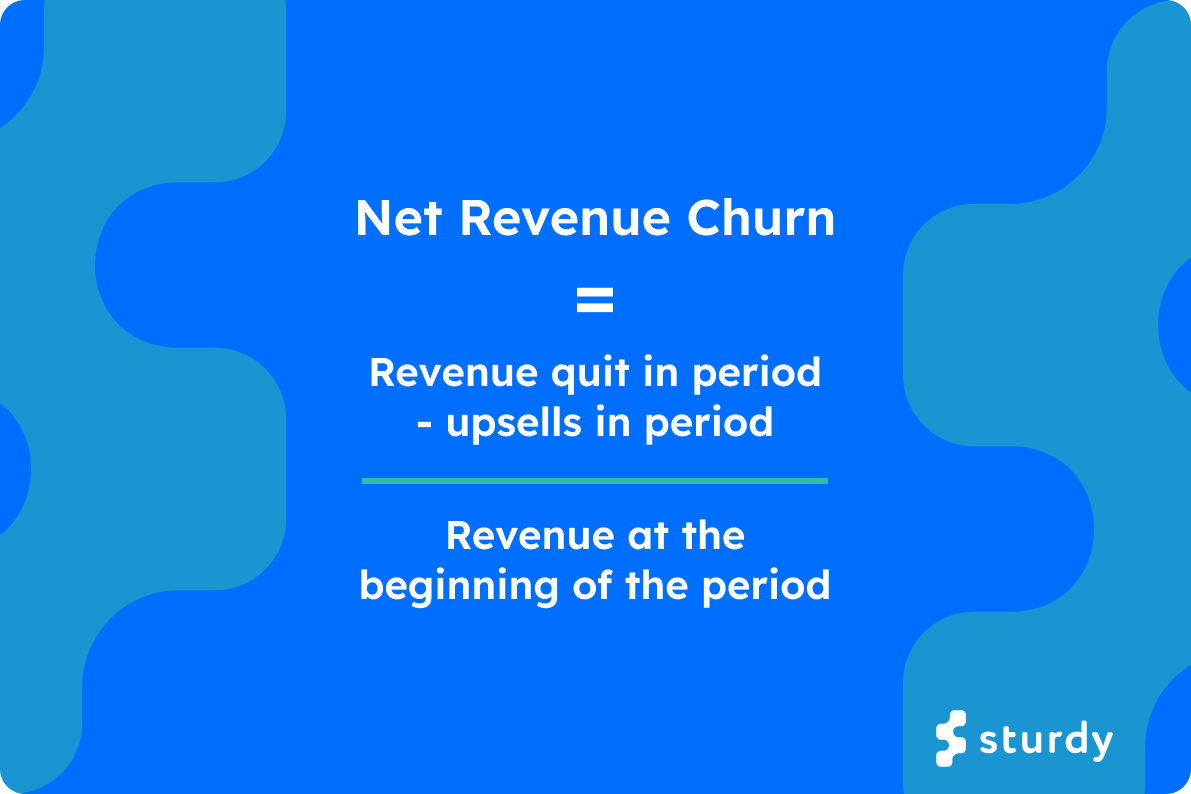
Net Churn Rate
Net churn rate considers both customer and revenue churn. However, it also includes new customers and expansion revenue acquired in a certain period. Expansion revenue is the additional revenue you generate from existing customers through upsells, cross-sells, or add-ons. That’s why net revenue churn gives an overall picture of how much business is being gained or lost in a given time frame.
A month has passed since those two customers, and 14% of gross MRR was lost. Saas.io is currently at $172,000 MRR in November, as no additional sales have been made. Unfortunately, November has also seen $12,000 in contract losses. Luckily for Saas.io, a few existing customers have upgraded their plans, generating an additional $10,000 in revenue. Your boss asks you what the net churn rate for November is. First, you must subtract the customer upgrade revenue from the revenue lost in downgrades and cancellations. Then, divide that number by the revenue at the beginning of November.
1. Total revenue at the beginning of a period: $172,000
2. Net revenue lost in period: $12,000 - $10,000 = $2,000
3. Net Churn Rate = $2,000/$172,000 = 1.1%

Leaky Bucket Equation
At the beginning of this post, we noted that four types of churn could be measured. That isn’t entirely true, so here’s a bit of a bonus round. SaaS angel investor, Dave Kellogg argues that the leaky bucket equation “should always be the first four lines of any SaaS company’s financial statements.” Kellogg continues, “I conceptualize SaaS companies as leaky buckets full of annual recurring revenue (ARR). Every time period, the sales organization pours more ARR into the bucket, and the customer success (CS) organization tries to prevent water from leaking out”.
Kellogg defines the leaky bucket equation as “Starting ARR + new ARR - churn ARR = ending ARR”.
If we apply this to our Saas.io example, we can determine that the starting ARR in the fourth quarter (Q4) of 2022 was roughly $400,000. The new ARR in Q4 ‘22 was $56,000, and the Churn ARR in that same time period was $45,000. In other words:
1. Total starting ARR: $400,000
2. New ARR: $56,000 & Churn ARR: $45,000
3. Ending ARR = $400,000 + $54,000 - $45,000 = $409,000
Churn is an important metric to track for any SaaS company, as it can be used to identify trends, measure loyalty, and assess the effectiveness of customer retention strategies. Calculating churn rates can help companies identify which customers are more likely to leave and which types of customers are the most valuable. By understanding churn, businesses can take steps to improve customer retention and keep their business running smoothly.
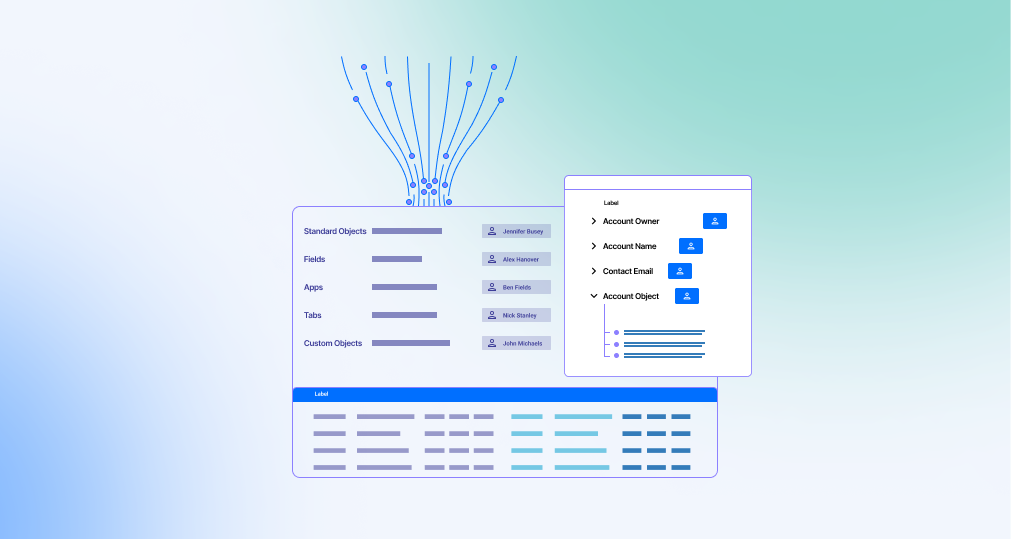
Sturdy PX: Automatic Product Insights from Unstructured Data
We’ve learned from our own operational experiences as entrepreneurs, product owners, revenue leaders, advisors, and board members that for SaaS companies to remain competitive, customers must be at the center of product and go-to-market decisions. Every team needs to optimize to create end-user value. To understand what really creates end-user value, you have to listen to customers — closely. Unfortunately, listening to customers is harder than it sounds.
The volume and velocity of data that businesses are generating is mindblowing. Ironically, most of this data is unstructured and trapped in emails, support tickets, Slack, call transcripts, user communities, and survey results. Most product teams struggle to grasp this valuable “dark data” at scale. Some are starting to experiment with AI to make sense of user interactions. This has introduced two new big problems: getting data out of all these sources and the murkiness of the data itself. This is why we create Sturdy PX for product-oriented teams.
My guess is that forward-looking teams trying to use AI are running into the same big issues that we had to solve to get Sturdy in the wild — mainly data transformation. We’ve seen this called data munging. Yuck. Whatever you call it, data prep tasks are time-consuming and tedious. We’ve learned that teams spend an enormous amount of time on manual data wrangling, which slows access to the valuable stuff - the analysis and, ultimately, the insights! About 70% of our tech is dedicated to automating data preparation tasks. For example, most companies collect data in inconsistent data formats across multiple modes/data sources. Sturdy standardizes the data formats, cleans the data, and synthesizes it with structured data like CRM data.
Leveraging AI, we're transforming traditional product research by empowering anyone on a product team with self-service access to the unsolicited, unbiased, and unabridged voice of the user. Sure, surveys, prototype tests, focus groups, etc., are still in the mix. They should be. But we’ve found that getting a consistent stream of accurate, categorized, product and user-centric insights really scratches that itch that we’ve had as product people.
For a limited time, we’re offering Sturdy PX to qualified product teams for free. It’s easy to get started, and the not-free version is pretty darn affordable, even on a tight budget. Get Sturdy here.
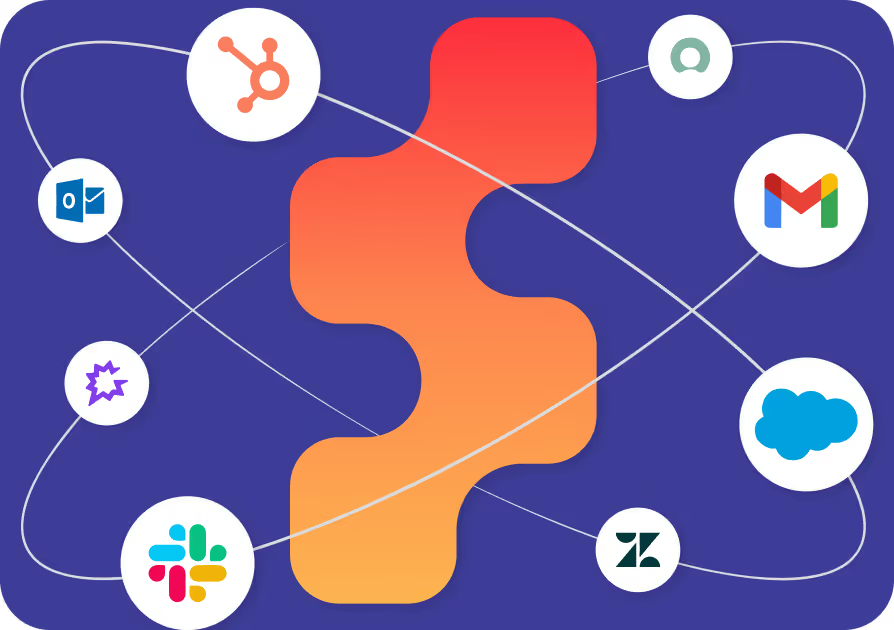
Leveraging Unstructured Data: How Business Leaders Can Harness the Power of AI
Introduction:
In today's digital age, the sheer volume of data generated by businesses is staggering. Ironically, most of this data is unstructured and trapped in things like emails, support tickets, and phone calls. Until now, this meant that the only way to extract valuable insights was by using manual labor to categorize them.
This is where the power of Artificial Intelligence (AI) comes into play. By harnessing AI, business leaders can unlock the hidden potential of unstructured data and gain a competitive edge. In this blog post, we will explore how business leaders can effectively leverage AI to extract valuable insights from unstructured data and drive innovation.
Understanding Unstructured Data:
Unstructured data refers to any information that lacks a predefined data model or organization. It includes text documents, social media posts, images, audio, videos, and more. Unstructured data is generated in abundance from various sources such as customer feedback, emails, surveys, social media platforms, and help desk interactions. The true value of unstructured data lies in its ability to reveal patterns, sentiments, and trends that can shape business strategies.
AI and Unstructured Data: Extract, Diagnose, Proact:
Artificial Intelligence, particularly techniques such as natural language processing (NLP) and deep learning, can process and analyze unstructured data with remarkable accuracy. By utilizing AI, business leaders can transform this seemingly chaotic mass of unstructured data into actionable insights.
Information Extraction:
- First, AI removes the ”manual labor tax” associated with leveraging unstructured data. AI efficiently extracts relevant information from unstructured text data, at scale, for a fraction of the cost of manual processing. Text mining techniques, including entity recognition, sentiment analysis, keyword extraction, and topic modeling, can be used to identify critical insights buried within vast amounts of unstructured text. This information can be invaluable for market research, competitive analysis, and trend forecasting.
Knowledge Diagnostics:
- The next step after extraction is leveraging this data to diagnose risks and opportunities. AI converts unstructured data into a powerful diagnostic tool. Unstructured data sources like customer emails and chat transcripts contain valuable information about individual products and processes. For example, a business leader may realize that just one feature is causing the majority of customer unhappiness. They might realize that a certain Account Representative is very good at improving sentiment. The possibilities for improving our businesses are almost endless.
Proactivity and Prediction:
- The “holy grail” of unstructured data is leveraging this information and knowledge to proact on and predict future events. By analyzing historical unstructured data, leaders can identify issues and monitor them going forward. For example, data might reveal that customers are more likely to cancel within 6 months of having a leadership change event. Not only will AI help leaders identify this warning sign, but by analyzing unstructured data in real-time, it will warn leaders and provide them with the opportunity to save revenue.
In the era of big data, unstructured data holds immense untapped potential. Business leaders who harness the power of AI can gain a competitive advantage by extracting valuable insights from this wealth of unstructured information. From sentiment analysis and text mining to predictive analytics, AI techniques provide the means to unlock the hidden value within unstructured data. By embracing AI and leveraging unstructured data, business leaders can make more informed decisions, drive innovation, and stay ahead in an increasingly data-driven world.

AI is Transforming Product Management
Product management has evolved significantly over the years, adapting to customer expectations, increased competition, and technological advancements. What was once a fragmented and operational role is now one of the most strategic and cross-functional disciplines in many organizations. Modern product management has two key goals; delivering customer value and, subsequently, driving business growth.
In the past, product managers were often hardened SMEs relying on previous experiences and assumptions. However, in recent years, subject matter expertise has become less critical as the focus has shifted to better understanding a wide variety of users. Product managers now emphasize understanding customer needs, conducting user research, and gathering feedback to inform feature prioritization and, more importantly, the desired value-driven outcomes for customers.
These days, PMs have a massive amount of data at their disposal, enabling experimentation and validation. This iterative mindset helps mitigate risks, validate hypotheses, and optimize product features based on user feedback. And, to that end, user feedback is the key — the currency of modern product management feedback is what makes or breaks a product.
Traditional user feedback methods are a miss
Traditionally, user feedback has been gathered through surveys and customer interviews. Surveys are emailed to customers to gather feedback. Pretty straightforward. Customer interviews allow PMs to dig deeper into customer motivations, pain points, and specific use cases.
What do traditional product research methods have in common? They are labor-intensive, often expensive, and time-consuming, requiring reliance on other teams to complete. And, maybe the biggest drawback is that the data and insights generated are typically from a small subset of the product’s user base.
Welcome to the AI-era
AI-powered platforms are making it possible to sift through data using natural language processing (NLP) and machine learning algorithms to quickly analyze large amounts of customer-generated information like email, tickets, call transcripts, and more. These data sets have been nearly impossible to access in the past because of their unstructured nature. AI-based tools can search for patterns and recognize key signals that might be difficult and even impossible for humans to spot, especially at scale.
AI is catapulting PM teams into a new era by enabling them to quickly and accurately identify trends in user preferences and behaviors related to specific feature requests, common product defects, and areas the most prone to user confusion.
Additionally, AI-based platforms analyze vast amounts of data in real-time, helping product managers iterate and experiment faster while reducing costs and reliance on other teams. With the help of AI, teams can gain valuable, unbiased insights into their products more efficiently and more effectively than ever before.
Real use case examples are maturing
For example, product teams at HireEZ, the award-winning outbound recruiting solution, use Sturdy to slice and dice real user feedback like feature requests, confusion, and product friction by product mix, segments, and cohorts to understand better how to maintain product-market fit across their customer base.
Teams at MP, a leading provider of innovative HR technology and managed HR services, no longer rely solely on surveys and interviews to understand customers better. They use the real voice of the customer from email and support tickets to better identify opportunities to improve their offerings.
It’s no surprise that AI is transforming product management. The function was poised for evolution. AI is now simply accelerating more teams to become even more customer-focused, data-driven, collaborative, and iterative. Product managers are embracing digital transformation, agile methodologies, and a customer-centric approach to navigate complex market dynamics and deliver innovative products.

Your employees spend most of their workday searching for information and moving data from one place to another
In today's world, the power of AI is undeniable and, in many cases, is yet unknown. Businesses are leveraging this technology to increase their productivity and efficiency in ways that were never before possible. From semantic search to content generation, AI has enabled teams across the globe to work smarter, faster, and more effectively than ever before. But what really takes AI-powered productivity to the next level is converging information. Zeya Yan and Kristina Shen contend,
To date, generative AI applications have overwhelmingly focused on the divergence of information. That is, they create new content based on a set of instructions. In Wave 2, we believe we will see more applications of AI to converge information…While Wave 1 has created some value at the application layer, we believe Wave 2 will bring a step function change.”
In other words, this synthesis or convergence of information promises to revolutionize how businesses operate. In this blog post, we'll explore how much time teams are currently wasting with mundane tasks and how Synthesis AI can ameliorate that.

Before we do that, let’s first define what Synthesis or Applied AI is. In simple terms, it is the use of artificial intelligence (AI) in a practical context. It involves using AI algorithms and techniques to solve real-world problems or create new products or services by synthesizing vast volumes of data into insights. Examples include self-driving cars, facial recognition software, natural language processing, and machine learning. Applied AI can help businesses save time and money by automating routine tasks and providing better insights into customer behavior. For example, Sturdy is an Applied AI vendor using several AI techniques to help businesses understand and act on customer and prospect interactions more effectively.
Let’s take a deeper look at how Applied AI can power the productivity of your team. We’ll begin by taking a close look at the standard American work week. According to a 2023 Zippia study, the average American adult works 38.7 hours weekly. That is roughly 8 hours a day during the standard work week. There seems to be plenty of time to accomplish meaningful work, but there’s a catch. Workers spend more than half of their workday searching for information and doing manual data entry. A 2022 Coveo report found that “the average employee spends 3.6 hours daily searching for information.” The report highlights an increase in one hour per day over the last year, a “trend heading in the wrong direction.” Imagine the impact that has on your business. To keep the math simple, if you have 100 employees working 262 days a year, that’s nearly 100,000 hours of wasted time a year. And as the old adage goes, time is money.

But it doesn’t end there. Your employees spend nearly as much time simply moving data from one place to another as they are searching for information. A 2021 Zapier study concluded,
76% of respondents said they spend 1-3 hours a day simply moving data from one place to another. Additionally, 73% of workers spend 1-3 hours just trying to find information or a particular document.”
Put that into perspective for one moment. That means your workforce is potentially spending up to 75% of their time looking for information or moving data from one location to another. Thanks to AI, it doesn’t need to be this way.
.avif)
For example, Sturdy uses Applied AI to make data entry and searching for information unnecessary. Sturdy collects all unstructured interactions with your prospects and customers—the stuff stuck in various silos—emails, chats, tickets, call transcripts, etc. It cleans it up, synthesizes it with different data sources, and gets it into one searchable system every team will use. And let’s be honest, your business will never generate less data than it does now. With Sturdy, the dark data trapped in your business finds the people, systems, and teams that need it most without requiring data entry. Now organizations can route cancellation insights to account managers—surface unbiased feature requests to product teams—send bug reports to the engineering team, and more. Sturdy automatically delivers the insights to answer the “why?” and “what next?” to the teams and systems that need it most. Yesterday you searched for information. With Sturdy, it will find you.





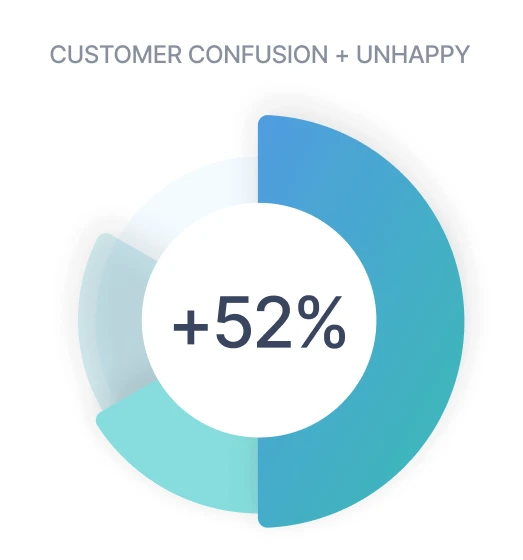
